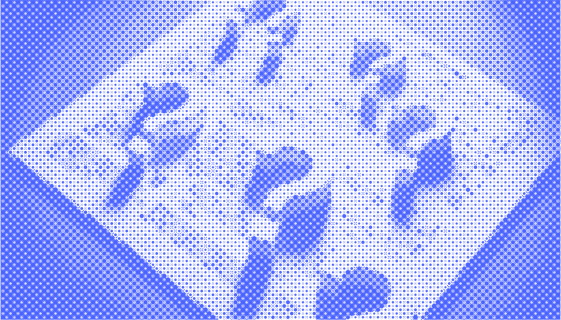
FraudGPT: How AI is—and isn't—revolutionizing financial crime

In the six months since ChatGPT was released, worldwide interest in artificial intelligence has tripled, reflected everywhere from watercooler discussions to comedy podcasts to businesses reimagining their futures. It's easy to understand this culture-wide fascination: constellations of tools and services built on machine learning seem equally capable of working with words, images, and sounds to produce convincing results. But as companies like OpenAI look to fine-tune their already astonishing offerings, everyone from New York Times journalists and world-renowned thinkers are also raising an important question: Is AI dangerous?
In the world of financial crime, yes: AI that is both increasingly sophisticated and available is making fraud and money laundering schemes more effective. Not only are bad actors evading traditional fraud detection and prevention efforts, the relatively low effort these tech-enabled scams require make them possible on an industrial scale. So what exactly does AI crime look like, and how are we to fight the darker side of today's AI boom?
APP: A well-oiled fraud machine
At least at first, crime will get a boost by simply plugging AI outputs into tried-and-true fraud techniques. The technique that has been finding the most traction in the age of instant, mobile online banking is authorized push payment (APP) fraud, which has already been wreaking havoc. Zelle, a payment service that conducts instant transfers for the likes of Bank of America and Wells Fargo, reported 190,000 cases of APP fraud leading to $213 million in losses in just 2021 and the first half of 2022. APP has become such an issue that regulators and governments are demanding action, and payments firms will soon have no choice but to act to counter it. So even before AI comes into the picture, it's important to understand how this already-popular approach works.
APP scams typically happen in three or four parts:
1. A scammer poses as a legitimate person or business and convinces their mark to send a sum of money to a bank account. This account appears legitimate but is, of course, controlled by the fraudster.
2. The funds rapidly hop from this first account to many other "money mule" accounts, covering or at least complicating its tracks and making up the second part of the scam.
3. Next, the money is extracted from the banking system as cash.
4. Finally (but optionally), the illicit funds can be re-injected into the banking system, taking already tainted funds through traditional money laundering steps of placement, layering, and integration.
In some ways this is the perfect fusion of both fraud and money laundering in that it is effective, hard to trace, and blurs the lines between teams handling fraud and anti-money laundering (AML).
How AI works as a fraud supercharger
For the devious, it might already be clear where AI can improve and automate APP fraud. For the rest of us, let's look at a few of the points in the process where fraudsters might get a real boost from technology, specifically generative AI, the label broadly applied to AI that creates new text, images, video, audio, code, or synthetic data.
Generated scenarios
When it comes to step one—targeting and reaching out to victims—creating realistic personas and communications convincing enough to motivate someone to willingly send their cash has never been easier. For example, fraudsters may use AI to impersonate customer service representatives from legitimate organizations. Imagine, for example, an official-sounding email from your cell phone company demanding payment. The sender's address may be expertly spoofed, but so too may be a link to a "customer service" chat, which in reality is simply a chatbot set up to "verify" that the request for payment is very real. ChatGPT can create such emails in seconds.
Generated identities
AI can also contribute to the second part of APP scams, wherein a string of money mules distance the victim's money from the initial receiving account to the scammer's final account. As a primary part of opening a bank or payment account, onboarding processes request a number of different types of personal information like ID cards, bank statements, payslips, or utility bills. It's easy enough to steal, buy, or create passable forgeries of these, but in recent years "liveness checks" have served as a last line of defense. These require photos in specific poses or even brief video chats with an onboarding specialist to verify that the person in the supporting documentation is the person opening that account at that very moment in time.
While deepfakes, videos trained to look and act like a real, usually notable, person, are highly publicized and already being put to use by fraudsters, generative AI accomplishes the same goal of bypassing liveness checks with far less effort. Rather than training to replicate a real and presumably verifiable person, generative AI allows convincing identities to rise up more or less out of nothing but a few sufficiently detailed prompts, complete with the ability to move and talk.
It's this low bar to entry that is the real threat of generated identities: it's simple to make an essentially unlimited number of them. Paired with convincing documentation, vulnerable systems can be fertile ground for the creation of an equally unlimited number of accounts under the control of an enterprising criminal wanting to scale up their operations.
In short, generative AI's strength is making content. Creating a flood of altered images, documents, and even entire identities is now an easy lift; when deployed in truly large numbers, it becomes inevitable that customers and onboarding processes will be fooled. Old safeguards like manual reviews and automations unable to piece together different parts of fraudulent identities will start to be overwhelmed.
Weaknesses of AI crime, or, Where to start fighting back
But as wary as we should all be of generative AI applied to crime, all is not lost. Shortcomings exist and will likely remain even as these AI tools continue to be developed. The main places where we can gain a foothold are in details and context.
Details
A really striking example came with the latest update to the Midjourney image generator. Looking at the photo below, it probably seems to most of us like a fairly standard photo of a woman in a kimono.
But as Twitter user @Ninetail_foxQ pointed out, to anyone with a bit of background knowledge, red flags were immediately apparent. From the construction of the kimono to the cultural significance of how it is wrapped, a trained eye immediately clocks that no real person would or could be the subject of such a photo. It's a fake, given away by unrealistic details. One human did spot this, but it can't be certain that others would. Or that they would do so 100% of the time. Or that they would do so consistently for 8 hours a day amid hundreds of similar such photos, some of which are real and some of which are AI-generated.
The problem becomes apparent, then, when it comes to far more subtle changes on far more detailed documents coming in at a much faster rate. Relying on humans to reliably identify a single changed number on one bank statement out of a thousand applying for new accounts is setting yourself up for failure when the goal is to take in as many (ideally legitimate) customers as possible with as little friction as possible.
Context
Essentially, where generative AI is going to supercharge schemes like APP fraud is in enabling more scams at greater rates. The goal will be to confront an onboarding process with more identities than it's built to deflect, with enough passing through that an army of fraudulent accounts stands ready to receive and process fraudulent funds. But this can be their undoing as well: the more generated identities, the more they start revealing patterns in the way they interact with financial systems. This is why context is the real solution.
Say a potential money mule account is caught trying to onboard, a classic case of forged or maybe even real documents and liveness checks trying to portray a fraudulent identity. Every aspect of that account can now be mined to catch other accounts lying in wait but sharing similar attributes. If a group of accounts used the same kinds of docs (or the same documents, full stop), were created within the same limited time frame, or show rhythmic patterns of trade with the same external entities, these and many more can become clear red flags of associated accounts flying under the radar or waiting to be activated.
Another example comes when a bad actor begins extracting their take from the digital payments infrastructure into the physical realm. Efficient ways of taking their cash with them are usually a priority—for instance, withdrawing from the same handful of accounts during consecutive ATM visits as seen below. This can be a clear clue in a chain that can be followed back and combined with other traits to tie together fraud networks in action.
If one were to look at these accounts and transactions in isolation to try to suss out their validity, it's a losing game. But by taking everything from onboarding to extraction—how someone is applying for an account, how they're using it, and how that compares to the wider customer base—into account simultaneously, patterns of documentation, identity components, and behaviors give away the game even for if someone has used generative AI to do the heavy lifting.
Fight fire with fire
Luckily, those on the right side of the law can also use AI to exploit these weaknesses in scammers' AI-driven tactics. Because, while humans can identify individual anomalies, creating coherent and adaptive models of identities, behaviors, and transactions that bring context to generated identities and entire payments networks is something AI is far more skilled at doing quickly and consistently.
AI can, for example, examine documents on a metadata level in a way humans can't, equally identifying most subtle changes or seemingly legitimate documents that have in fact been created out of whole cloth. Perhaps an even greater strength is AI's ability to note when a legitimate—albeit stolen—document has been reused over and over, or when numerous attempts to create accounts all stem from a suspiciously similar location. Stopping money mules at the point of attempted creation in this way isn't just the most effective way of stopping the APP fraud that relies on them, it's an inexpensive and scalable way to plug the gaps between traditional document and liveness checks.
Transaction-wise, AI can keep track of countless criteria all at once in a way that humans simply can't. By putting these together, patterns in money flows as well as anomalies in existing patterns can make money laundering and other suspicious activities clear as day. Money mule networks can expose themselves, repetition in ill-gotten funds being reintroduced into the financial system, and even a victim sending an out-of-the-ordinary payment can all be pointed out and even stopped in real time.
The takeaway is simple: ways of moving money and those who rely on them are more threatened than ever by a revolution in financial crime committed by those capitalizing on the dangers of AI. But companies who recognize this—and who recognize the vulnerabilities in this new age of fraud and money laundering—also have a chance to protect their customers better than ever by using the very same technologies.