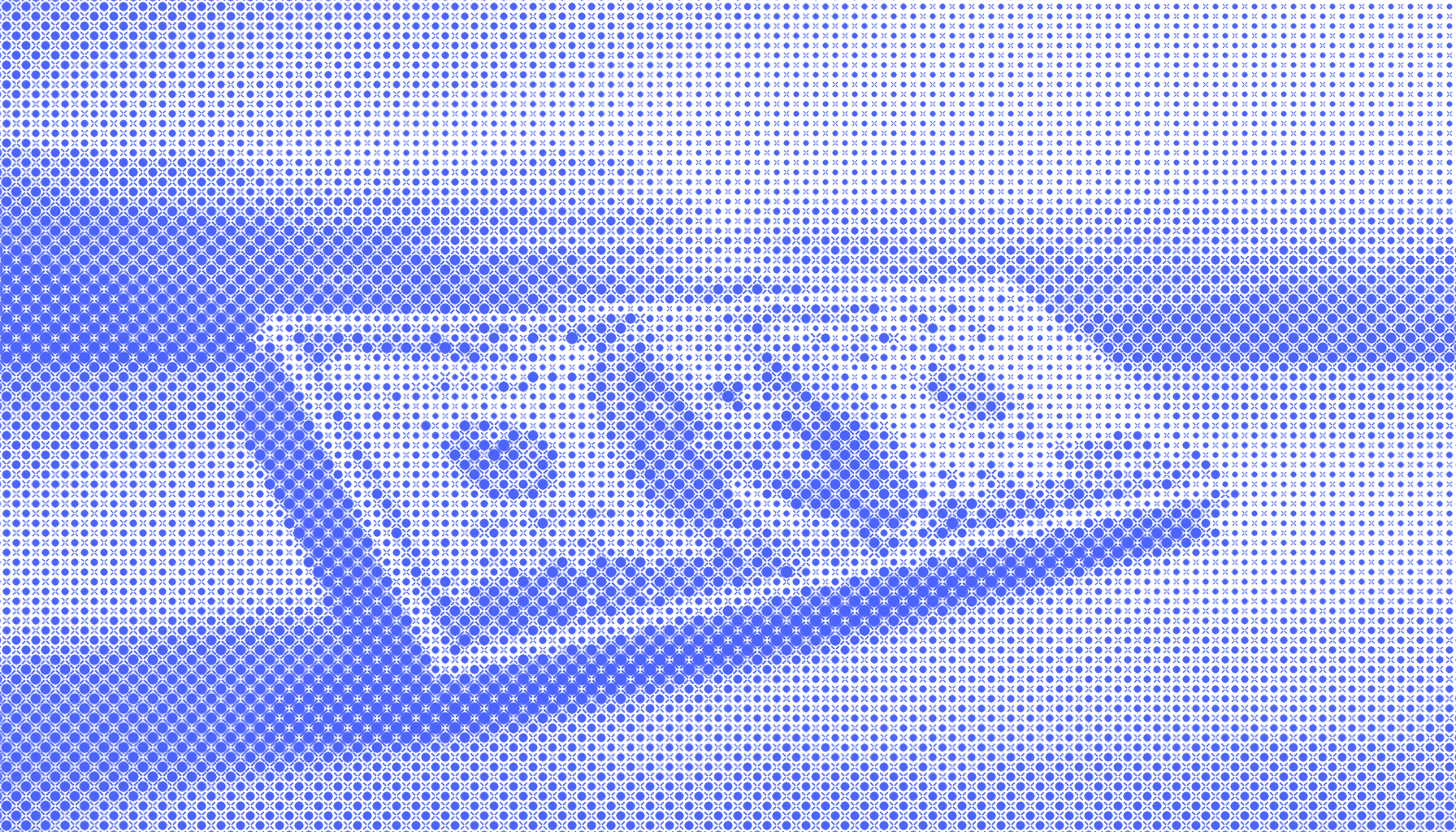
Ensemble modeling: A game-changer in financial crime detection
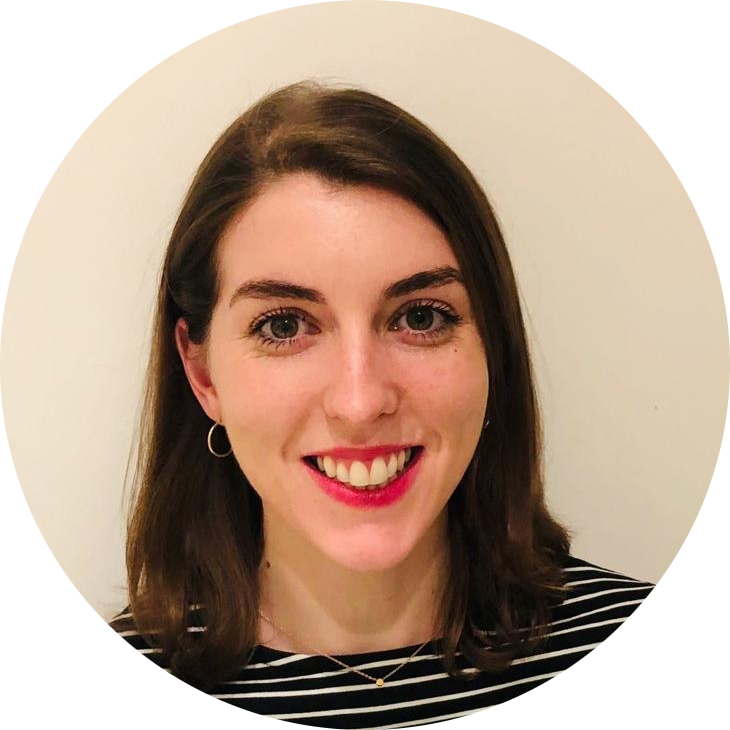
More than ever before, financial institutions and fintech companies are recognizing the need to adopt cutting-edge AI technologies to combat financial crimes, and transaction monitoring is a critical aspect of these anti-financial crime (AFC) efforts. However, traditional rules-based transaction monitoring systems which rely on largely binary triggers to detect pre-defined criminal behaviors generate a huge amount of false positives and repeat alerts. This well known limitation results in investigators sifting through vast volumes of noise to confirm true suspicious activities. In addition to being time-consuming, this approach means some crimes never get reported in time — or at all — with predictable consequences.
This is where AI and machine learning come into play — these capabilities enable organizations to more effectively process large volumes of data, detect undefined patterns, and significantly improve the efficiency of their transaction monitoring systems. By effectively applying machine learning, organizations can detect known risks with greater speed and accuracy while also increasing their detection of novel, previously unknown criminal activities.
However, implementing machine learning can be easier said than done, as it’s sometimes difficult to know where to get started.
What is ensemble modeling — and why should organizations apply it to decisions in AFC?
Ensemble modeling is a game-changing approach that’s largely still in its early days of adoption within the anti-financial crime landscape. In transaction monitoring, there are a wide range of factors that need to be accounted for when assessing behavior — multiple data points must be considered in order to ensure effective detection. It’s extremely difficult to take account of all of these factors in a single model.
To resolve this issue, ensemble modeling allows the use of multiple, smaller models that are highly focused on a specific sub-problem. It’s the outcome of these specialized models that determine whether the behavior is suspicious. This approach is especially effective for transaction monitoring because it offers a comprehensive view of the complex data that’s typical in financial services.
While it’s conventionally accepted to seek out experts to get the best information, it’s often proven that a crowd of laymen can supply better aggregated knowledge. As author and New Yorker business columnist James Surowiecki puts it, “When our imperfect judgments are aggregated in the right way, our collective intelligence is often excellent.”
This logic is at the heart of ensemble modeling: While conventional reasoning may suggest building the biggest model that can encapsulate the world, it’s the combined performance of smaller, less perfect models that gets the best result.
Top benefits of ensemble modeling
Our team has found that ensemble modeling offers the most practical approach to detection: Each of the models that it comprises offers complementary information about the behaviors, and when these are combined, they improve the performance of detecting a wider range of unusual behaviors while also promoting higher accuracy and a reduced number of false positives.
Ensemble models allow organizations to analyze different components of transactions and focus on specific aspects of the task at hand, whether it’s examining the size of a transaction, its location, or the nature of the counterparties.
Financial institutions and fintech companies can reap the following benefits delivered by the ensemble model:
- Effective tuning: Ensemble modeling makes use of a greater number of less complex models, which allows greater control over tuning such models. Organizations can easily adjust parameters and thresholds of individual models, which results in the more accurate, adaptable detection of financial crimes.
- Identifying sophisticated financial criminals: Ensemble modeling allows banks to identify not only individual suspicious activities but also clusters of criminal behavior. Weak evidence related to one person alone may not lead to an escalation. However, with the help of AI, organizations can leverage even the faintest patterns in their data to detect and disrupt clusters of criminal activity and adversarial actors looking to exploit and manipulate their systems. At Resistant AI, we apply this at two levels: On a detector level (multiple models involved in a single detector) as well as a system level (multiple detectors applying to our customers’ transactions).
- Enhancing explainability: Explainability is vital for financial crime analysts and investigators. Ensemble modeling ensures that the decisions made by AI aren’t just accurate — they’re also easily explainable and can be properly interpreted by stakeholders.
- Reducing false positives: The use of multiple models analyzing the behavior from different perspectives results in more robust decision making and therefore reduces the false positives generated, ensuring high-precision detection.
Explaining AI for investigator effectiveness
As mentioned above, explainability in AI is not merely a regulatory requirement — it’s a critical component of analyst and investigator efficiency. Simply put, financial crime investigators need transparency over the factors that determine AI-driven outcomes, as having an understandable explanation for why a transaction was flagged as suspicious is essential for effective investigations. Without having proper explanations, investigators may struggle to initiate their investigations, which can lead to inefficiencies and delays.
“When we say something is important we can tell you why,” Resistant AI CEO Martin Rehak said in an interview with insideBIGDATA. “We can tell you which indicators point to this. We can essentially write a report for each finding that says this should be a high risk because of these factors.”
On a related note, the concept of interpretability is often used in the context of explainability. It implies that stakeholders can comprehend the main drivers of a model-driven decision. Ensemble modeling inherently supports interpretability, as it involves combining simpler, more explainable models. As a result, these models provide interpretable outputs, making it clear why a particular transaction was flagged as high-risk.
In an ensemble, individual models assess just one factor in a transaction and provide explanations in human-readable language. This approach enhances both interpretability and transparency, and importantly, it aids investigators in understanding the reasons for alert generation (especially when transactions are blocked or held for further investigation). This level of detail is key in building trust and ensuring effective decision-making.
How financial institutions can get started with machine learning
Using important takeaways from our team’s engagements with customers, we’ve established a reliable, five-step process for organizations that are interested in implementing machine learning:
- Define core objectives: First, it’s necessary to clearly define the objectives of implementing AI in transaction monitoring. Set realistic goals that align with the organization's risk mitigation strategy, such reduction of manual processing times, faster time to react to new threats, and increased detection of fraud or other financial crimes.
- Set realistic implementation timelines: With proper planning, AI implementation doesn’t need to be overly costly or time-consuming. Carefully plan out the implementation process and be sure to allow for contingencies.
- Identify day-to-day users: Determine which team members will interface with the AI solution and involve them in the implementation process — this will ensure that key stakeholders have a well-rounded understanding of the system's capabilities and limitations.
- Map implementation to growth goals: Consider how AI-based transaction monitoring will support the organization's growth plans. On this point, it’s important to think ahead — in other words, leaders and decision-makers should assess how AI can assist in prioritizing work as transaction volumes scale.
AI is no longer simply a buzzword — it’s an umbrella term for many actionable programs that financial institutions and fintech companies can implement today.
As organizations across the globe continue to face evolving threats, the adoption of AI and machine learning approaches like ensemble modeling is essential for staying ahead in the battle against financial crime. By properly understanding and implementing this approach, organizations can protect their operations and comply with regulations — all while maintaining the trust of their customers and stakeholders.